We have seen statistical modeling and machine learning used in financial services for a while now. Often by the larger banks, lenders, and collection agencies. They do this to reduce risk with credit scoring and fraud detection in large-scale, broad applications. But what if you could do the same thing but on a smaller scale? What if that could make the operational side of your organisation more efficient and effective? Lastly, is fully automated features in machine learning a possibility with the right fintech solution?
Best known for risk management
In the financial industry, they frequently use statistical models to make better predictions that can improve efforts in managing risk. Using models to calculate and rank credit scores and other risk factors can help an organisation make better decisions. Decisions such as whether to lend money and under which conditions.
Machine learning improves the predictive power of statistical models because they can be continuously ‘trained’ with new data. The ability to better predict outcomes with probability calculations also makes it quicker to notice ‘unusual’ situations. This is the reason machine learning is so useful. It greatly helps in the fight against money laundering and other forms of financial fraud.
A learning machine is any device whose actions are influenced by past experience.
Nils John Nilsson
Applying machine learning to operations
The large-scale analysis of big data is an expensive process, however. You need teams of data analysts to study huge amounts of data to build the right models. Then the models still need to be continuously updated to maintain and improve the accuracy of their predictions. Interesting for those broad applications that are important to the business, but much too expensive and complex to apply to the day-to-day operations to make individual processes and activities ‘smart.’
Until now.
Automated features in machine learning
With our fintech platform (Aptic ARC), we discovered that if you have the right IT architecture, it is actually possible to add fully automated machine learning features. These can make the operational workflows both more effective and efficient. We’ve built an innovative extractor for our platform. It can automatically collect data and simultaneously normalise it. This can then be used directly in predictive statistical models – all without the need for a team of data analysts.
The digitalisation of that process makes innovating with machine learning possible on a much smaller scale. Consequently, it becomes much more attractive for the operational side of financial services. On our platform, it means that you can apply models to every step and action in the entire operational process (the workflow) and use multiple models at once. It is even possible to apply multiple models to automatically choose between complete workflows.
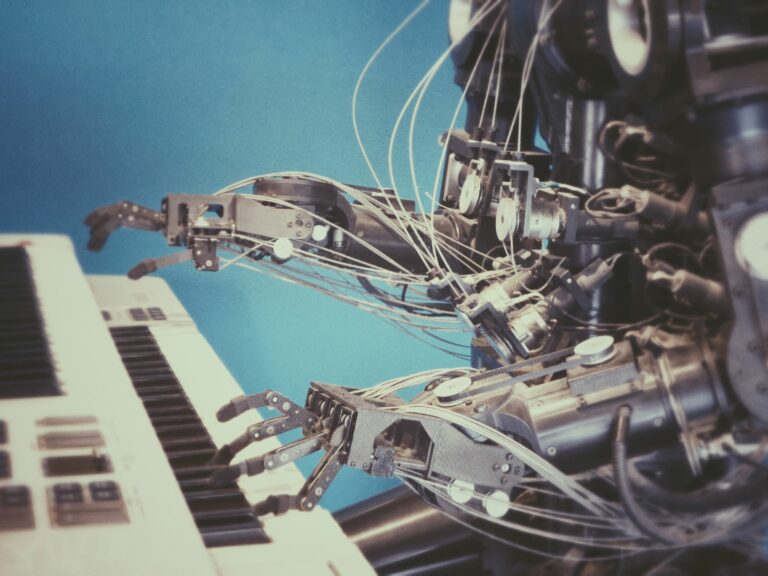
An example of optimised fintech operations
Imagine that you want to find a better way to reduce past due receivables. (Who doesn’t?) Your normal accounts receivable workflow has a rule set for when unpaid amounts reach 28 days past the due date. The standard action here is to send a reminder letter through the post. You wonder if there might be a more effective action that increases your chance of getting the desired response (immediate payment).
There are multiple options that might work. Should the letter be friendlier? Would email or text messages work better? And of course, not every solution will be equally effective for every customer. Are there differences in effectiveness for different age groups or different genders?
The automated machine learning function can test all these factors efficiently. It can do so with each of the various options to select the options that offer the best possible outcomes. It includes data for other factors that might affect the outcomes, such as the size of the unpaid amount, payment frequency, history of late payments, and other debts and open account balances.
The system tests all these various factors randomly against the available options for actions (standard letter, friendlier letter, SMS, etc.) each time the action point for a reminder occurs in the workflow. It then automatically collects data on the results. Running this test phase for a while, a few months in this case, ‘teaches’ the system something valuable. It teaches which combinations of factors and actions give the highest probability of achieving the desired outcome. After the training period, the resulting model can be used to automatically select the best option based on the combination of factors in each individual case.
Endless optimisation
A reminder letter is just one example. You can test and implement models for every single step and every action point in the entire workflow. For example, you could also create multiple models to optimise the number of days between various actions, all running in parallel and allowing you to optimise your operations endlessly. It is even possible to create models that can use specified criteria to automate the choice between different options for the whole workflow.
Let’s take another example from accounts receivable collections. Imagine that you want to know if, and in what cases, a reminder by text message two days in advance would increase the rate of payment from new accounts. To test this, you can simply create two separate workflows: Workflow A with the advance warning via SMS and Workflow B without it. Then run a test period in which you use Workflow A half of the time and Workflow B the other half. After the training, the system will be able to choose on its own, which complete workflow to follow to get the best results.
Digitalisation brings AI within reach
Using this automated method of machine learning on the operational side of financial services makes it easy to fully digitalise daily processes and make them much more efficient. All without compromising on effectiveness.
This functionality puts AI within reach for other financial services providers than just the big banks. Factoring, leasing, collections, and business and consumer credit can all benefit. The automated process means you can implement statistical models without the need for large teams of analysts processing enormous amounts of data.
Our solution makes automated features in machine learning possible
ARC is Aptic’s advanced fintech platform for factoring, lending, debt collection, and other types of financial services. Want to know more? Feel free to contact us; hello@aptic.net
Interested in learning more about machine learning and other technological innovations in financial servicing? Download our latest whitepaper